Common Scenarios:
- Overpayments:
- This occurs when more money is paid than owed.
- Resolution involves recovering the excess amount.
- Underpayments:
- This happens when less money is paid than owed.
- Resolution requires paying the remaining balance.
Key Actions and Considerations:
- Immediate Notification:
- As soon as the error is detected, it's crucial to notify the relevant parties (e.g., vendor, customer, bank).
- Accurate Record-Keeping:
- Maintain detailed records of the original payment, the error, and all subsequent actions taken.
- Communication:
- Clear and concise communication is essential to ensure everyone understands the situation and the steps being taken to correct it.
- Recovery or Payment:
- Overpayments:
- Request a refund from the recipient.
- If necessary, follow up with formal requests or legal action.
- Underpayments:
- Make the additional payment as soon as possible.
- Provide clear documentation of the corrected payment.
- Prevention:
- Implement stronger internal controls to prevent future errors.
- Utilize accounting software with built-in error detection.
- Conduct regular audits of payment processes.
- Bank involvement:
- If the error is related to a bank transfer, contact your bank immediately. They may be able to help recover the funds, especially if the error was recent.
- Legal recourse:
- In situations where the other party is unwilling to cooperate, legal action may be necessary.
This highlights another important category in AP errors: correct payments for wrong amounts. This is a nuanced problem that differs from both duplicates and overpayments.
In these cases, the payment itself is processed correctly (to the right vendor, with proper approval), but the underlying invoice amount is wrong from the start. The payment accurately reflects what was invoiced, but the invoice itself doesn't match what should have been charged.
Common causes include:
- Vendors billing at outdated price points not matching current contracts
- Charging for quantities different from what was actually delivered
- Including items that weren't ordered or received
- Misapplying discount terms or promotional pricing
- Mathematical errors on the vendor side
- Incorrect application of taxes or surcharges
- Contract terms being misinterpreted by the vendor
These errors are particularly challenging to detect because they often pass through standard AP controls. Three-way matching might not catch them if receiving documents don't properly verify quantities or qualities. Standard duplicate payment detection won't find them since they're not duplicates.
Prevention typically requires:
- Regular contract review and vendor price list validation
- Detailed receiving processes with careful quantity and quality inspection
- Periodic vendor audits to verify pricing compliance
- Training procurement staff on contract terms and pricing structures
This type of error highlights why comprehensive AP controls need to extend beyond just payment processing to include the entire procure-to-pay cycle.
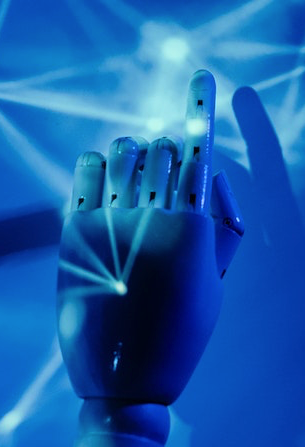
Would Incorrect Amounts Outwit Automation and AI?
Yes, incorrect amounts can indeed outwit automation and AI—especially if the discrepancies are subtle or systematic rather than blatant errors. While automation and AI are powerful tools for detecting anomalies, they have limitations that can be exploited or overlooked, depending on how they are set up.
๐ก How Incorrect Amounts Can Evade Detection by Automation and AI:
1. Subtle Discrepancies:
- Small variances in amounts, such as rounding errors or minor adjustments, might not trigger alerts.
- Fraudulent invoices with amounts close to expected values may pass through automated checks.
2. Pattern Mimicking:
- Consistent but incorrect amounts that mimic historical patterns may not be flagged as anomalies.
- If an AI model is trained on incomplete or biased data, it might learn the wrong "normal" behavior.
3. Data Formatting Tricks:
- Changing the presentation of amounts (e.g., $1,000 as 1000.00 or 1k) can confuse automation scripts.
- Using different currencies without proper conversion can lead to acceptance of incorrect payments.
4. Vendor Master Data Issues:
- Duplicate vendor records with slight differences can allow incorrect amounts to be paid without detection.
- Automation rules that rely on vendor names or IDs may not recognize inconsistent data entries.
5. Complex Invoice Structures:
- Invoices with multiple line items, taxes, discounts, and freight charges can create scenarios where the total amount is incorrect, but individual components appear valid.
- AI might struggle with invoices containing credits, returns, or partial payments, where the correct amount is not straightforward.
6. Anomaly Overfitting:
- If the AI model is trained on a narrow set of data, it might fail to generalize and miss new types of discrepancies.
- Rule-based automation can only detect predefined scenarios, leaving unusual errors unnoticed.
๐ฆ Strategies to Prevent Incorrect Amounts from Outwitting AI and Automation:
๐ง 1. Use Multi-Layered Validation:
- Combine rule-based automation with AI anomaly detection to cover both expected and unexpected scenarios.
- Introduce thresholds and tolerance levels to flag small discrepancies for manual review.
๐ 2. Employ Fuzzy Matching Techniques:
- Use fuzzy logic to identify invoices with similar but not exact values, which can help detect subtle differences.
- Implement AI models that analyze historical payment data to spot patterns of minor yet consistent overpayments.
๐ 3. Advanced Anomaly Detection Models:
- Leverage Machine Learning (ML) techniques like clustering and outlier detection (e.g., Isolation Forests, Autoencoders) to find irregularities.
- Use predictive analytics to model expected payment amounts based on purchase orders, historical transactions, and contract terms.
๐ 4. Introduce Manual Review for Edge Cases:
- Set up workflows where invoices with unexpected amounts are automatically sent to finance or AP teams for review.
- Create a 'gray zone' where payments within certain margins require human approval.
๐งฎ 5. Improve Data Quality:
- Regularly cleanse vendor data to eliminate duplicates and inconsistencies.
- Standardize invoice formats and data entry practices to reduce interpretation errors.
๐ ๏ธ 6. Set Dynamic Rules and Alerts:
- Implement dynamic rule sets that adapt to changing business conditions, such as seasonal price changes or new vendor contracts.
- Configure alerts for payments that deviate from historical averages or expected ranges.
๐ก Our Advice:
Train your AI models not only on correct payments but also on historical mistakes, allowing the system to learn from past errors and improve detection accuracy.
It's true that incorrect amounts can pose significant challenges for automation and AI systems. While AI is powerful, it's not infallible, and certain types of errors can indeed "outwit" it. Here's a breakdown:
Challenges Incorrect Amounts Pose to AI:
- Data Dependency:
- AI algorithms, especially machine learning models, rely heavily on accurate and consistent data. If the data they're trained on or receive as input contains incorrect amounts, the output will also be flawed. "Garbage in, garbage out" is a key concept.
- Contextual Understanding:
- While AI can perform complex calculations, it may struggle to understand the context behind an incorrect amount. For example, it might flag an unusually high payment, but it may not be able to determine whether it's a genuine error or a legitimate transaction.
- Anomaly Detection Limitations:
- AI can detect anomalies, but it needs to be trained on what "normal" looks like. If incorrect amounts are frequent or varied, AI may have difficulty distinguishing between genuine anomalies and routine errors.
- Human Oversight Still Needed:
- Even with advanced AI, human oversight is crucial. Humans can apply critical thinking and contextual awareness to investigate potential errors that AI might miss.
- "Automation Bias"
- There is a tendancy for humans to trust automated systems too much. So if a AI system produces a incorrect number, humans may be more likely to accept it than if a human produced the same incorrect number.
How to Mitigate the Risks:
- Data Quality Control:
- Implement robust data quality checks to minimize errors in input data.
- Rule-Based Systems:
- Combine AI with rule-based systems that can enforce specific business rules and flag transactions that violate those rules.
- Human-in-the-Loop:
- Incorporate human review into critical processes, especially for high-value transactions or those with a high risk of error.
- Continuous Monitoring and Auditing:
- Regularly monitor AI performance and conduct audits to identify and address any errors or biases.
Remember:
- While AI can significantly improve accuracy, it's not a silver bullet.
- Incorrect amounts can create challenges, but these can be mitigated through careful data management and human oversight.
- It is important to remember that AI is a tool, and like all tools, it has its limitations.
Great question. Incorrect amounts on otherwise valid invoices present a significant challenge for automation and AI systems, but they don't necessarily outwit them completely.
Basic automation tools would likely miss these errors because they typically focus on matching what's on the invoice to what's in the system rather than validating whether the underlying amounts themselves are correct. If an invoice matches a purchase order in format and reference numbers but contains incorrect pricing, simple automation would likely process it without detecting the error.
More sophisticated AI solutions, however, can be designed to catch these issues by:
- Learning historical pricing patterns for specific vendors and products
- Comparing current invoice pricing against contract terms stored in the system
- Flagging statistical outliers (e.g., a 20% price increase when typical increases are 2-3%)
- Analyzing trends across multiple invoices from the same vendor
- Comparing unit prices across similar vendors for the same products
The effectiveness depends on:
- The quality and completeness of your historical data
- How well your AI system is trained on your specific pricing agreements
- Whether contract terms are digitized and accessible to the AI
- The sophistication of your AI model's anomaly detection capabilities
The most effective approach tends to be a hybrid model where AI flags potential pricing anomalies for human review rather than attempting to make definitive determinations on its own. This combines AI's ability to process large volumes of data with human judgment to interpret complex pricing terms and agreements.
Organizations with mature AI implementations are increasingly able to catch these errors, but it requires more sophisticated training and implementation than basic duplicate detection.
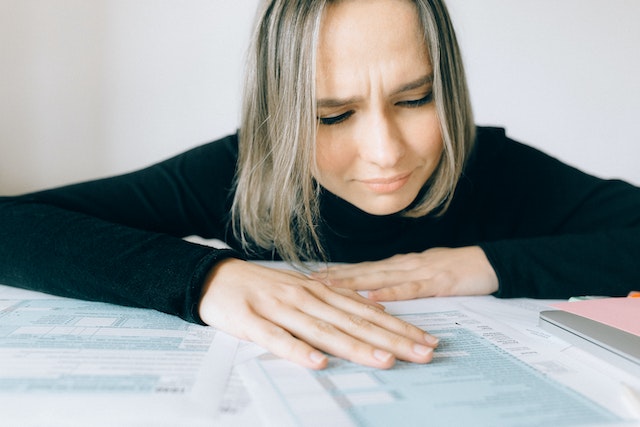
Custom Train an AP AI?
This is a very important and realistic point about the practicalities of implementing AI in specific departments like Accounts Payable (AP). It's usually the reality that a single AP department is unlikely to have the resources and data required for custom AI training. Here's a breakdown of why:
Challenges Faced by Individual AP Departments:
- Data Volume and Variety:
- AI, especially machine learning, thrives on vast datasets. An AP department, while processing many invoices, might not have the sheer volume and variety of data needed for robust AI training.
- AI models need diverse data to account for the many variations in invoice formats, vendor practices, and potential errors.
- Data Quality:
- AI models are only as good as the data they're trained on. AP data can often be messy, with inconsistencies and errors. Cleaning and preparing this data for AI training requires specialized skills and time.
- Technical Expertise:
- Developing and training AI models requires expertise in machine learning, data science, and software engineering. Most AP departments lack these skills in-house.
- Computational Resources:
- AI training can be computationally intensive, requiring powerful hardware and software. This can be costly and beyond the budget of a single department.
- Data Security and Privacy:
- AP data contains sensitive financial information. Ensuring the security and privacy of this data during AI training is crucial, and this adds complexity and cost.
- Model Maintenance:
- AI models are not static. They require ongoing maintenance and updates to remain accurate. A single department may struggle to provide this.
What This Means in Practice:
- Reliance on Vendor Solutions:
- Most AP departments will rely on AI-powered solutions provided by vendors. These vendors have the resources and expertise to develop and train AI models on large datasets.
- These solutions are often designed to be adaptable to different AP workflows and can be customized to some extent.
- Focus on Integration:
- AP departments should prioritize integrating AI solutions with their existing ERP and accounting systems. This allows for seamless data flow and efficient use of AI capabilities.
- Emphasis on Data Governance:
- Even if a department isn't training it's own AI, they still need to focus on good data governance. This will improve the performance of any AI that they are using.
Perhaps AI does really offer significant potential for AP automation, individual departments should focus on leveraging existing AI solutions and ensuring data quality, rather than attempting to build their own AI models from scratch. But custom training an AI model for Accounts Payable (AP) is not a straightforward task for a single department, especially without access to large datasets, specialized skills, and adequate resources. Many AP teams operate within broader finance departments, often with limited budgets and IT support, making the task of building and maintaining a custom AI solution challenging.
๐ฆ Key Challenges for a Single Department to Train an AP AI:
1. Limited Access to Data:
- Historical AP Data: Many organizations have data silos, preventing the AP department from accessing historical invoice and payment data needed to train AI.
- Insufficient Volume: AI models, particularly machine learning models, require large datasets to learn effectively. A single department may not generate enough data to provide reliable results.
- Data Diversity: An effective AI model needs exposure to a wide variety of scenarios (e.g., different invoice formats, varied vendors, currency exchanges, contract types). Smaller datasets might lead to overfitting, where the model works well on training data but fails in real-world scenarios.
2. Data Quality Issues:
- Incomplete Records: Missing invoice details, purchase orders, or payment confirmations can skew model training.
- Data Inconsistency: Variations in data entry formats, naming conventions, and coding practices reduce data usability.
3. Technical and Resource Constraints:
- Lack of Expertise: Building an AI model requires data scientists, machine learning engineers, and software developers, which smaller AP teams typically do not have.
- Budgetary Limitations: Custom AI development involves software costs, infrastructure, training, and ongoing maintenance, which might not be justifiable for a single department.
- Time Constraints: AP teams are often busy with day-to-day operations, leaving little time for AI experimentation or model training.
4. Integration and Implementation Barriers:
- ERP Systems: Many organizations use complex ERP systems (e.g., SAP, Oracle, Microsoft Dynamics) that may not easily integrate with custom AI models.
- Automation Pitfalls: Even if an AI model is developed, implementing it effectively requires integration with existing processes, automation tools, and reporting systems.
๐ ๏ธ Practical Alternatives to Custom AI Development for AP:
๐ก 1. Leverage Pre-built AI Tools:
- Use off-the-shelf AP automation software with built-in AI and machine learning capabilities, such as:
- Tipalti, AvidXchange, SAP Concur, Kofax, and Basware.
- These solutions often include:
- Automated invoice processing.
- Duplicate and overpayment detection.
- Machine learning models trained on industry-wide datasets.
๐ 2. Utilize ERP and Accounting Software Features:
- Many ERP systems offer AI-powered modules or add-ons for AP management.
- Examples include:
- Oracle Autonomous AP, which uses machine learning to detect anomalies.
- Microsoft Dynamics 365, with AI-driven insights for payment optimization.
๐ง 3. Consider SaaS Solutions with Embedded AI:
- Many Software as a Service (SaaS) platforms provide AI tools that are easy to integrate with existing systems:
- ApprovalMax: Automates approval workflows with AI-powered insights.
- GEP SMART: Uses AI for invoice management and overpayment detection.
๐ 4. Use Analytics and BI Tools:
- Apply Business Intelligence (BI) tools with pre-built AI models, such as:
- Power BI, Tableau, or Qlik Sense, which can help visualize payment trends and identify anomalies.
๐ 5. Collaborate with Vendors:
- Many vendors offering AP automation tools provide pre-trained AI models and can offer customization as part of their service packages.
- This approach allows AP teams to benefit from AI without needing to build custom models themselves.
๐ Approach for AP Teams with Limited Resources:
- Identify Specific Needs: Focus on a single pain point, such as duplicate payment detection or invoice matching, rather than building a broad AI solution.
- Choose a Scalable Solution: Start with pre-built tools that can scale up as needed.
- Implement in Phases: Test AI on smaller datasets or specific processes, then expand as confidence grows.
- Monitor & Optimize: Use the feedback loop to improve automation rules and fine-tune existing AI features rather than creating custom models.
๐ก Pro Tip:
Instead of training an in-house AI model, focus on establishing clean data practices and standardizing processes, which will not only improve current automation tools but also make your department AI-ready for future implementations.
Would you like recommendations for specific AP tools with AI features, or do you need help evaluating your current systems to see if AI integration is possible? ๐